Sunday, January 12, 2025
Enterprise Knowledge Graph, practical applications in the customer support world
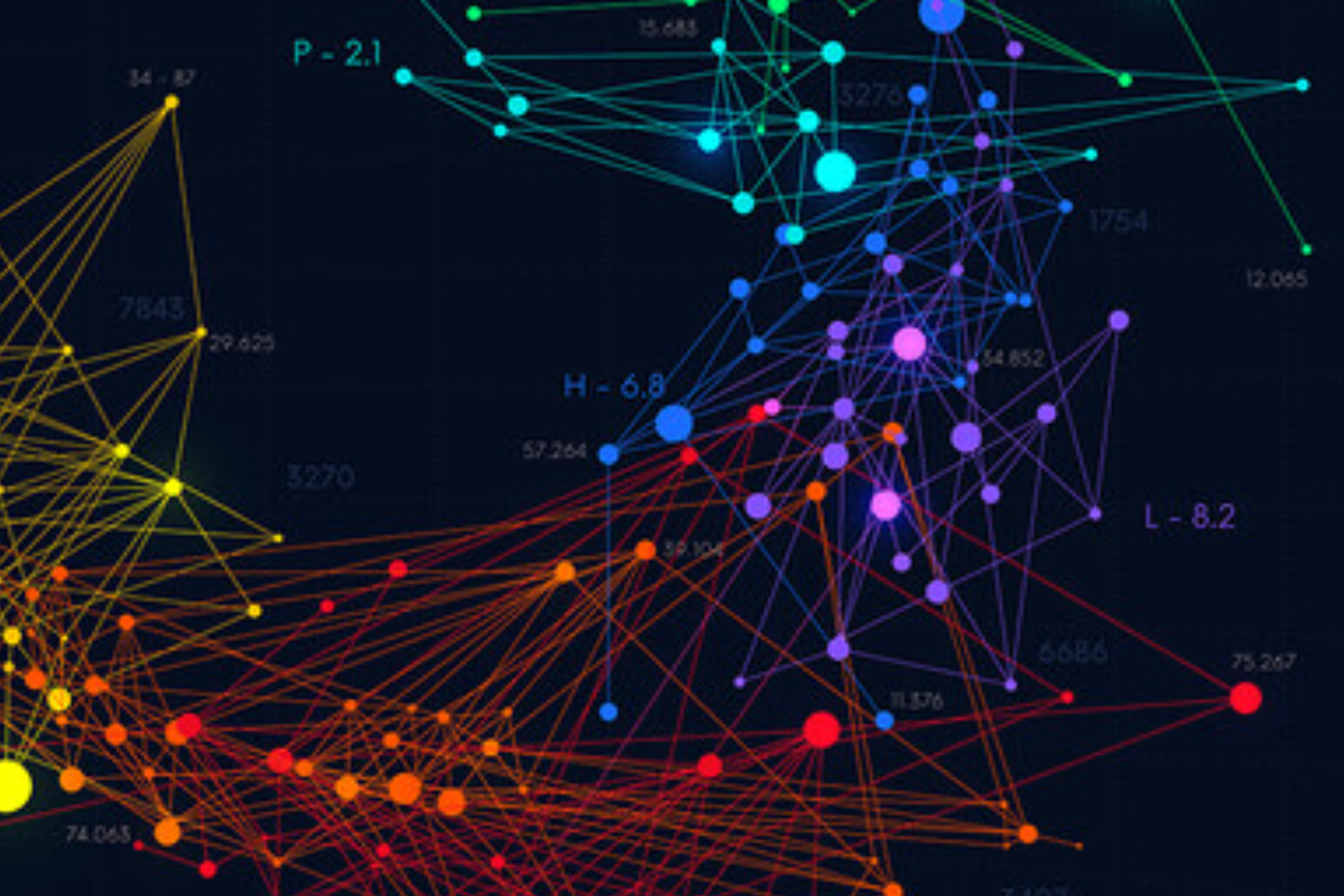
After 15 years, building, maintaining and supporting end customer service applications, I came to a realization that a comprehensive knowledge management solution is a must for any organization with a customer interaction surface that spans a few interactions per day.
From seeing hundreds of inquires per day coming though a major government organization for the rules to establish a new company, to tens of individuals coming in person to the court offices just to know their hearing date, my understanding for the complexity of customer support started to mature.
One quick realization was that this complexity actual arise from a few challenges…
- Information privacy varies a lot: from public rules and regulations , to customer individual records that require authentication, to public tender information that is still protected by a paywall !
- Constant changes and updates: Governmental, organizational and other procedures and regulations are constantly changing, let alone customer records and transactions.
- User experience is tricky!: each individual customer has their own channel preferences (web, phone, in-person, social… etc) and each channel has its own constraints.
Here is a quick example: a customer interacting with an AI agent in the baking space though voice notes over whatsapp, asking the AI agent (using voice notes!!) about their individual record while a fallback to a human agent and the entire conversation is expected to complete with an FTR (first time resolution and AHT (average handling rate) under 1 minute!!
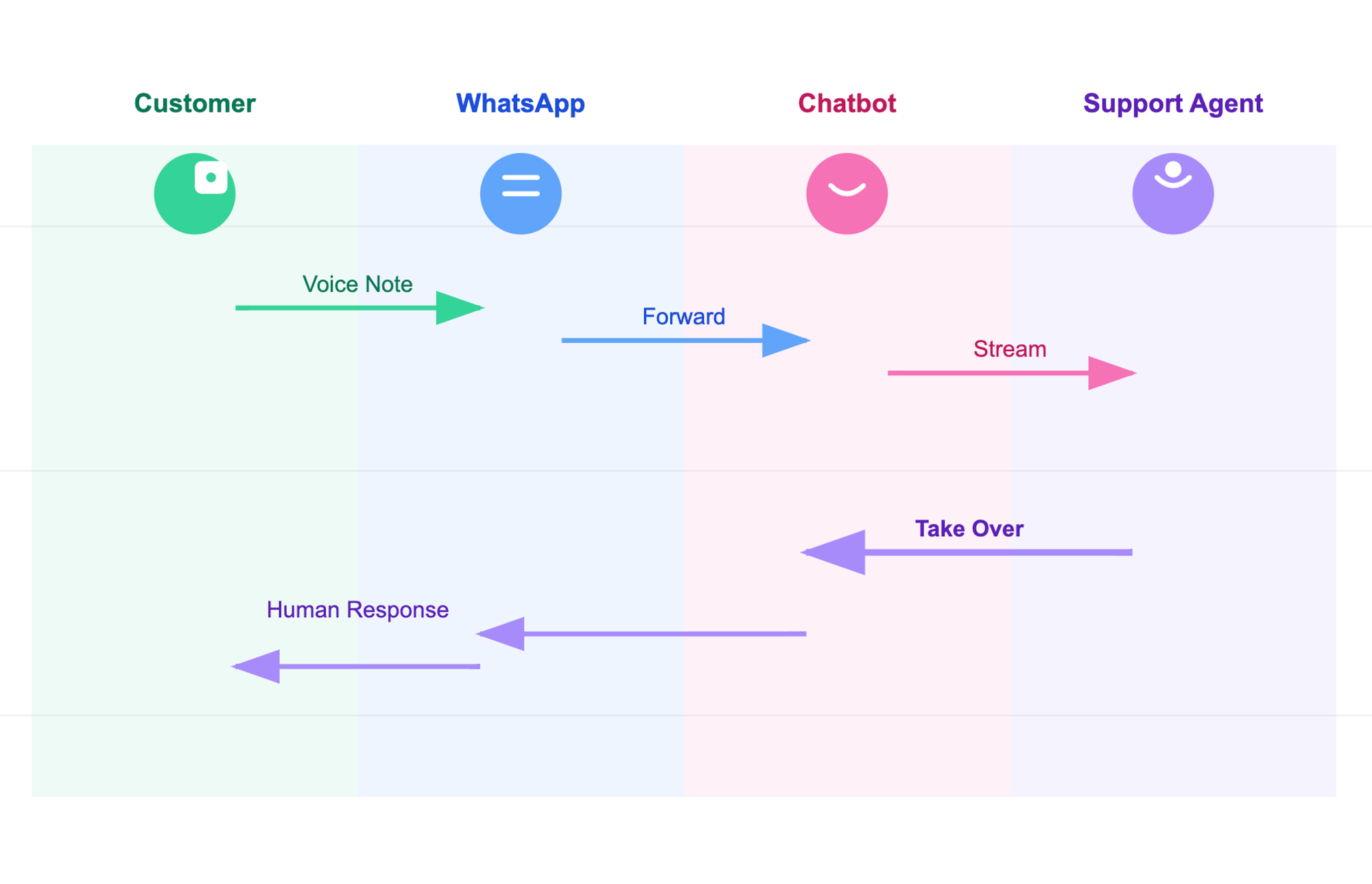
Knowledge graphs to the rescue
Understanding the basics
Essentially, a knowledge graph is a flexible data structure constructed based on relationships between data points. For example, in a banking context, a knowledge graph might connect a customer entity to their accounts, transactions, and personal information. A simple relationship could be "John Smith (customer) owns Savings Account #12345 (account)". These connections allow for complex queries and insights, such as identifying all customers with high-value accounts who have recently made international transfers.
Knowledge graphs excel at representing and linking diverse information types, making them ideal for customer support scenarios where AI and human agents need to quickly access and understand interconnected data. They can seamlessly integrate structured data (like account balances) with unstructured data (such as customer service call transcripts), providing a holistic view of the customer and their interactions with the organization.
How to construct a knowledge graph for customer support?
In my experience, after building multiple knowledge graphs for customers in various sectors, I found that constructing a knowledge graph for customer support isn't as complex as it might seem. For example, if i’m going to build a knowledge graph for a customer in the banking sector, i would start by identifying key entities: customers, accounts, products, support interactions, in addition to public information like articles and guides for different procedures. These become our graph's nodes.
Next, we define relationships between these entities. For instance, "Customer owns Account," "Customer inquired about Product," or "Support Interaction resolved Customer Issue for a specific procedure." These relationships form the edges of our graph. To populate the graph, we integrate data from various sources: CRM systems, transaction databases, and even transcripts from customer service calls using natural language processing.
A critical step is enriching the graph with context. We can tag nodes with attributes like account balance, customer preferences, or common issues related to specific products. This context allows both AI and human agents to quickly grasp the full picture when assisting a customer. For example, when a customer calls about a declined transaction, the agent can immediately see their account status, recent activities, and any related issues, all in one view.
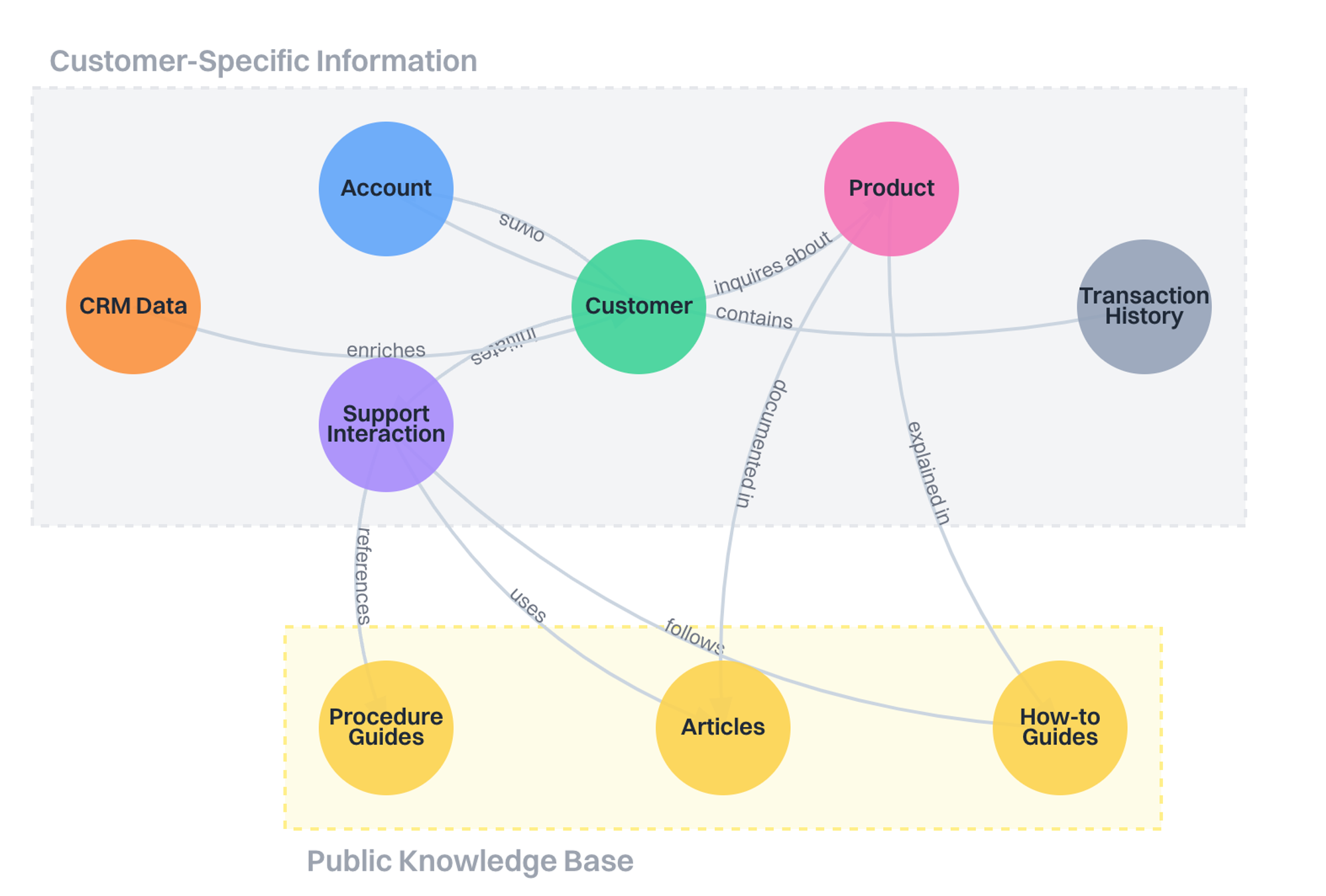
Bridging public knowledge and customer / transactional data
In my opinion, connecting generic information with individual customer records is where knowledge graphs truly shine in customer support. Let's take international transfers as an example. On one side, we have public information about how to make an international transfer - the process, required details, and general guidelines. On the other, we have a customer's specific transfer details and account information.
To bridge these, we create nodes in our knowledge graph for both the general process and the customer's specific transfer. We then establish relationships between these nodes. For instance, we might link the step "Provide recipient's IBAN" in the general process to the actual IBAN the customer used. This connection allows our support agents or AI to seamlessly move between explaining the process and addressing the customer's specific transfer, all within the same interface. It's this contextual linking that enables truly personalized, efficient support, reducing handling times and improving customer satisfaction.
Another benefit is easily linking customer support requests to a specific product (international transfer) and a specific support article (how to make an international transfer) which might indicate the need for an improvement for this product or support article.
Practical Applications of Knowledge Graphs in Customer Support
In my years of experience working with various organizations, I've seen firsthand how knowledge graphs can revolutionize customer support. They're not just theoretical concepts; they're practical tools that can significantly enhance customer interactions and streamline support processes. Let me share some real-world applications that I've found particularly effective.
Contextual Customer Support
One of the most impactful applications of knowledge graphs in customer support is providing real-time, personalized assistance. By integrating customer data, interaction history, and product information, support agents can access a complete customer 360 view instantly. For example, when a customer calls about a product issue, the knowledge graph can immediately present relevant information about their purchase history, previous interactions, and potential solutions based on similar cases.
This level of personalization extends to self-service options too. Imagine a customer portal that adapts its content based on the user's profile, presenting tailored FAQs, product recommendations, and support articles. This not only improves the customer experience but also reduces the workload on your support team by enabling more effective self-service.
Intelligent Query Routing and Response
In a large telecommunications company, the knowledge graph connects customer queries with historical resolution patterns. When a customer contacts support about their internet service, the system instantly maps their issue to similar past cases, considering factors like their service plan, location, and recent network incidents. This enables automated routing to the most qualified agent or, in many cases, provides immediate automated responses.
Dynamic documentation updates
Consider a government agency handling business registration procedures. Their knowledge graph automatically updates all related documentation when a regulation changes. For instance, when a new requirement for business registration was introduced, the data ingestion layer uses the graph to automatically identify all affected procedures, forms, and customer communications that needed updating. This eliminated the manual process of tracking down and updating hundreds of documents, reducing update errors by 90% and ensuring consistent information across all channels.
Conclusion: The Future of Customer Support is Connected
After exploring enterprise knowledge graphs in customer support, it's clear this technology isn't just another tool – if we combine it with the power of AI agents, it becomes a fundamental shift in how we manage and deliver customer service. Looking back at our initial challenges – varying information privacy, constant updates, and complex user experiences – knowledge graphs provide a unified solution that addresses all these pain points.
The beauty of knowledge graphs lies in their adaptability and ability to connect public knowledge with private customer data while maintaining strict privacy controls. Start small, perhaps with a single department or product line, and let the graph grow with the customer benefit. As AI continues to play a larger role in customer support, having a well-structured knowledge graph will become crucial for delivering truly intelligent, personalized support experiences.
The goal isn't just to store information – it's to make knowledge accessible, actionable, and relevant at the moment it's needed. In an era of rising customer expectations, this connected approach to knowledge management isn't just an advantage – it's a necessity.
Interested to see Knowledge Graphs in action? Sign up for our beta!